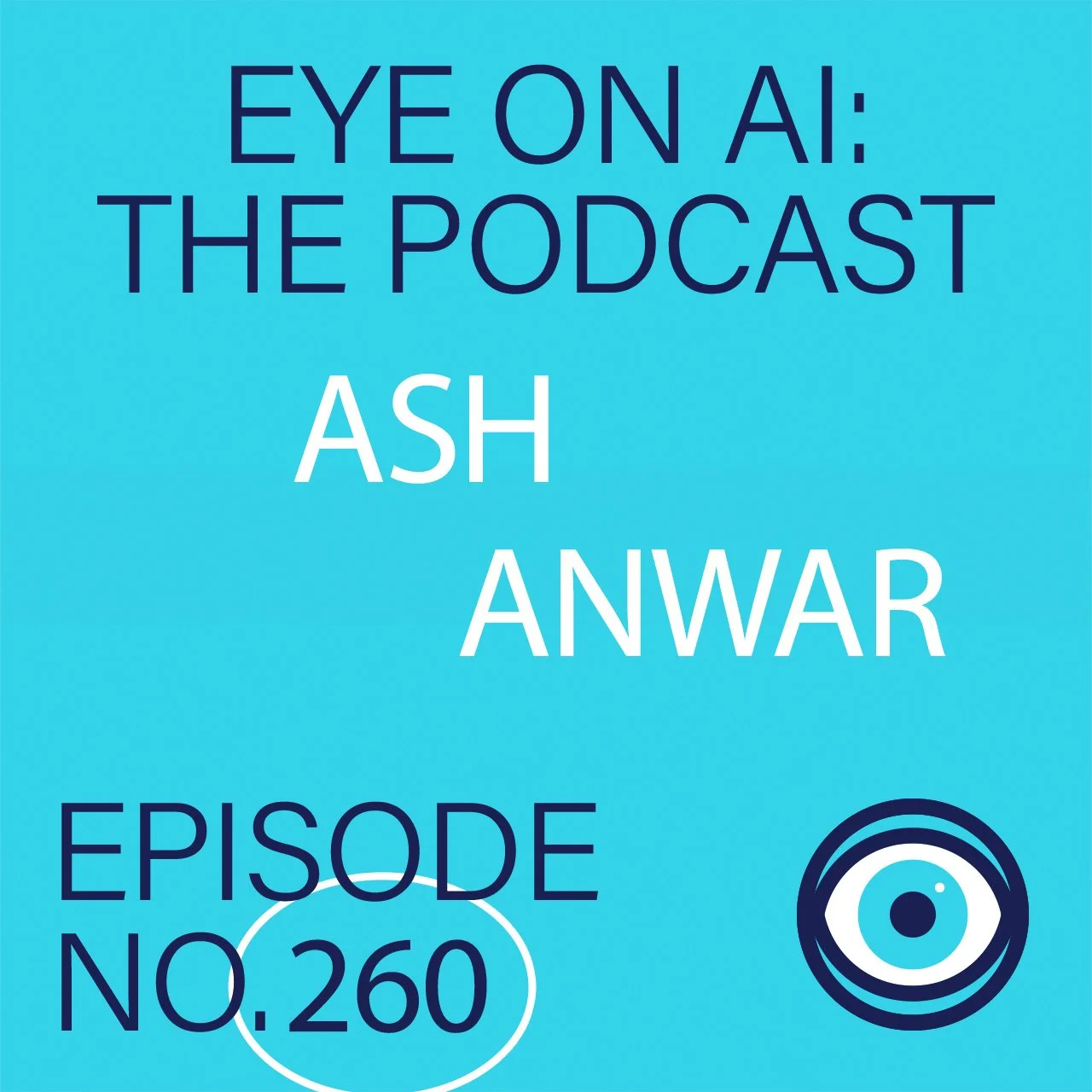
Ash Anwar, co-founder of Molecular You, explores how AI and advanced biomarker analysis are transforming how we detect and prevent disease. Ash breaks down how Molecular You is shifting healthcare from reactive treatment to proactive longevity.
Eye_On_AI_-_260_-_Audio.mp3: Audio automatically transcribed by Sonix
Eye_On_AI_-_260_-_Audio.mp3: this mp3 audio file was automatically transcribed by Sonix with the best speech-to-text algorithms. This transcript may contain errors.
Ash Anwar:
The datasets we started with are exactly what you said labeled datasets that usually accompany a lot of the published information too. So definitely the deep scientific literature, clinical literature. We're able to acquire the datasets of patients either from the authors or the publications that have used this, and a lot of biobanks will also have this information available as well. So being able to use that data as a basis to kind of develop the models and then also battle test this and see how accurate the models are at predicting disease, as well with validating through real patients.
Ash Anwar:
The research is to curate and identify novel biomarkers that we wanna incorporate into our platform. We are also constantly evolving the different disease areas and biological mechanisms that we're including into our platform as well.
Craig:
Build the future of multi-agent software with agency A-G-N-T-C-Y. The agency is an open source collective building the internet of agents. It's a collaboration layer where AI agents can discover, connect and work across frameworks. For developers, this means standardized agent discovery tools, seamless protocols for interagent communication and modular components to compose and scale multi-agent workflows. Join Crewai, langchain, llama, index, browserbase, cisco and dozens more. The agency is dropping code specs and services, no strings attached. Build with other engineers who care about high quality multi-agent software. Visit agencyorg and add your support. That's A-G-N-T-C-Y. Dot O-R-G. Join other engineers who care about building the internet of agents, so go ahead?
Ash Anwar:
Yeah, sure. So I'm Ash Anwar. I'm the co-founder and senior director of scientific research and development at Molecular U. My educational background is rooted in science and business, so I did my undergraduate degree at Simon Fraser University in molecular biology and biochemistry. Then I went to the University of British Columbia to do my graduate degree in experimental medicine, where I did research in neurological conditions like Alzheimer's disease, multiple sclerosis, and then I went on to Harvard Med School to do my research fellowship in ophthalmology. I was really interested in transitioning from neurology to vision disorders, so my research project at Harvard was around age-related macular degeneration, which is the leading cause of blindness in older adults.
Ash Anwar:
And once I was complete there in Boston, I decided to come back to my hometown, which was Vancouver, and look at opportunities to transition from academia into more of an industry scientific role.
Ash Anwar:
That's when I met Rob and he mentioned that we are starting up this company in experimental, in looking at translating scientific information and getting into the hands of individuals.
Ash Anwar:
And that's what my entire program in experimental medicine was all about to go from bench to bedside, as they call it, go from scientific research to the bedside. And so I got really passionate about that and joined the company got it off the ground and everything, and so I joined Molecular EU and that was back in 2015, I believe. And yeah, and you know we're bringing all that information together. I was really able to work on this information from the ground up and really help build that. And while I was at Molecular U, as I was growing in the company, growing the team and we were growing the organization, I saw that there was a need for translating not just the science information, but also understanding how science can play a business role in industry as well. So I also ended up doing my MBA from the University of British Columbia as well to help wrap up on that aspect and get that knowledge base of business too.
Craig:
enough data and enough powerful enough algorithms and compute to see what effect those biomarkers, or what those biomarkers can tell us about the emergence of disease and longevity. Is that right? And and I gotta say uh to listeners I interviewed eric topo, who's a well-known doctor in the ai space, uh, about a book he has coming out I think I mentioned to you, to you last time we spoke called uh, uh, super agers and uh, uh, it's. It's specifically about this, about, uh, that there exists this technology that can be applied to the data, uh, regarding these biomarkers, and that we should be able to predict, uh, you know, uh, the onset of, uh, the major, uh, major disease classes that limit longevity. And if you can predict them, then you can address them, even though we know how to address them anyway.
Craig:
But people don't necessarily follow doctor's advice without a deadline, so to speak, in a very real sense. So can you talk about so I'm going to run this episode back-to-back with the one about Eric, because you guys are doing precisely that Can you talk about how molecular you works? First of all?
Ash Anwar:
Yeah, absolutely. I think the premise is exactly what you mentioned. We are looking at the early indicators of disease. Traditional medicine, with the biomarkers that they're looking at, really is in this diagnostic classification. So what the physicians are looking at are the biomarkers that signal that disease and damage has already occurred. Symptomatically, the patient is exhibiting certain symptoms of the disease, basically, and so that's really, if you think about a flow flow chart of how disease works, that's really at the end, when things have created damage, something is wrong in the system. Then the patient goes to the physician and says, hey, I think I feel bad. They look at the biomarker and say, okay, you have, you know, this disease. Potentially I'm going to give you a medication to correct the, the factor that's that's happening, or the symptom. That's usually treatment of the symptoms that's occurring.
Ash Anwar:
What Molecular U is looking at is we're looking at the early stages of that disease, and this is where our bodies are actually quite resilient. So there might be damage occurring in our bodies, but our bodies are essentially maintaining what's known as homeostasis, maintaining a state of normality, as best as it can while the damage is still occurring. And over time what happens is this damage can accumulate. And if you think about like dominoes, like the first domino may start to fall, but it's only until you get to that last few dominoes where the entire thing collapses and then you are in the full throes of the disease. And so we're looking at those early root causes of the disease.
Ash Anwar:
This is where these biomarkers come into play and that's where not only are we getting an indication of the specific early indicators of the disease, but a lot of times diseases work through multiple different pathways so developing diabetes of the disease.
Ash Anwar:
But a lot of times diseases work through multiple different pathways, so developing diabetes or cardiovascular disease, for example. One individual may go down a certain biological pathway to develop that disease, but another individual might go through another set of biomarkers that leads down to the same diseases. And this is where, molecular, you looks at the combination of those biomarkers and is able to inform that okay, this is the path that potentially can lead to a risk of having that disease. And, as you mentioned, with the super agents, if we could look at those biomarkers that impact our longevity, impact our ability to maintain a disease-free life, we can actually target those during those early cases of damage and then reverse at that stage where things are very reversible and get us back on track to a healthy longevity state, rather than waiting until symptoms occur. And that's when you've had so much accumulation of damage, it becomes much, much harder to get back to a healthy state accumulation of damage, it becomes much, much harder to get back to a healthy state.
Craig:
Right, so? And the idea is that you track a patient over time. This is a longitudinal study where you would have a blood test annually and see how the biomarkers are changing. Is that right?
Ash Anwar:
Correct, correct. Yes, I mean that's and that's really powerful, because looking at how we uniquely change over time and how our biomarkers are dynamically changing with our environment you know the stresses we have in our environment, what we eat, what our exercise habits are, things like that that is all going to have a significant impact on our biomarkers, and being able to longitudinally track how we change and how the dynamicism of that works gives us an unparalleled resolution into how our bodies are trajectoring over the health landscape.
Craig:
Essentially, Right and presumably this is. You know you train the model and then you backtest it with known data to improve the model or optimize the model, fine tune the model. How effective has this process been in identifying, when you look back over time?
Ash Anwar:
been in identifying. When you look back over time, it's been very, very quite effective. Actually, I mean being able to retrospectively look at the data. When we understand the endpoints of where an individual has gone towards, we're able to essentially trace back the path that certain biomarkers have taken to go out of that normal range and we've been able to look at the different biological processes that have gone awry in the early stages. So having a model that enables us to predict a certain chronic disease, for instance, and then looking at data where the individual or the group of individuals has developed the disease data where the individual or the group of individuals has developed the disease enables us to be able to identify those different tracks not just one but multiple different tracks and at what stages these biomarkers have gone out of the normal ranges. And being able to feed that data back into the model has increased the accuracy in our predictive value of these models. So it's been a really boon for that.
Craig:
Yeah, and how many markers do you track?
Ash Anwar:
As of now, more than 250 biomarkers. So these biomarkers are the metabolites and proteins. So metabolites are essentially the chemical byproducts of the chemical reactions that occur in our bodies. So anytime we exercise or eat or have stress, if we're not sleeping very well, the chemical reactions in our body will result in these metabolites that were very dynamic and will change. And then also this other omic as well, which we'll analyze, which is the proteins and these are the structural and functional elements, is the proteins and these are the structural and functional elements of our body. So these are the things that make up our muscular skeletal system. Proteins are essentially a big part of the enzymatic components that enable these chemical reactions to occur as well. So it's 250 biomarkers plus right now and we're looking to increase to around 800 plus within this year as well, which will give us a wider panel.
Craig:
And you said that you only need a vial or two vials of blood to track. Is that possible? 800 biomarkers.
Ash Anwar:
Yes, yes, so it is a full vial of blood, just like you go to the doctor and they take one vial of blood. The technology we use is mass spec, so it enables us to be able to essentially use this sample and go through a high-throughput panel that enables us to analyze a lot of these biomarkers at the same time. So this is the process that gives us the ability to increase the number of biomarkers while still not exponentially increasing the amount of collection that we would need to do for the patient.
Craig:
And how frequently do you need to sample the blood to track changes in the biomarkers?
Ash Anwar:
Yeah, this is a really great question. I think it's a great question because the answer is it depends. It depends on the situation.
Ash Anwar:
Very often, I think a very common way of doing this longitudinal assessment really is an annual checkup.
Ash Anwar:
Very common way of doing this longitudinal assessment really is an annual checkup where the individual comes in and does essentially an annual test to look at where they are in terms of their health state and then in the next year they will go and do another test to see has there been changes? What is the delta of the changes of these risk trajectories that we're looking at in terms of these different chronic diseases that we're all put on? So that's, I would say, a very good default situation. However, there are a few I would say a few cases where if an individual is already been diagnosed with a certain disease, maybe they have diabetes already or they've had a cardiovascular event in the past, like a heart attack. That's when having a shorter or more increased frequency of testing will lead to the benefit of being able to track even more quicker changes that may occur and actually doing interventions even quickly, to have an even maximum benefit in those specific individuals, even quickly, to have even maximum benefit in those specific individuals.
Craig:
Yeah, you know, you showed a presentation when we first spoke and it had a case of a woman who you had followed for some time I don't remember how many years and in the visualization that you showed, you could see something was starting to happen around 2019. And I think by 2022,. There was clearly something wrong and she subsequently, or as a result, had a full body. I think it was a CT scan or an MRI, I'm not sure which. I think it was a CT scan or an MRI, I'm not sure which.
Ash Anwar:
Yeah, it was a CT scan.
Craig: 15:48
Yeah, and they identified a lesion on her pancreas. It turned out to be malignant, but it was stage one and so they were able to operate. I don't know if she had chemo or radiation, but that, I'm sure, resonates with a lot of listeners. I just had a friend die very suddenly and unexpectedly of pancreatic cancer, and one of the problems is that it's asymptomatic until it's stage four, and then it's too late to do anything, and then it's too late to do anything. So, yeah, can you talk about that? Was that unusual? How often are you able?
Ash Anwar:
Well, first of all, how many people are you tracking? We're tracking, we're talking about in the thousands of individuals right now, and exponentially increasing now that we've done our launch into the US market as well. So we have a lot of clinics on board and are deploying this test to their individuals, individual patients, as well. So it's you know, it's increasing a lot, the number of individuals we are tracking at the moment, and I'm, yeah, I'm very sorry to hear about your friend.
Ash Anwar:
I mean, cancer is a very devastating illness and very often, especially in the case of like pancreatic cancer, it is very hidden until the very end where the cancer becomes really aggressive and really there isn't a lot of interventions that can occur. We were actually quite lucky with this patient as well. I mean, she's been with us for a very long time and has been doing these annual testing as well, more so from a curiosity standpoint and wanting to be in the space of longevity. She's 60 years old, so she really wanted to think about like, oh, how do I have a good quality of life well, into my later years as well, and I want to make sure that I optimize my health, I want to make sure that I understand what is the lifestyle that I need to maintain to keep this high quality of life, or even optimize this, the quality of life that she has and it's.
Ash Anwar:
It was during the, the time of COVID actually, where she started complaining about, you know, fatigue, excessive fatigue, not being able to work as effectively as she she did, shortness of breath, things like that. So she approached her doctor around you know, some of these symptoms and and they were very nonspecific and they weren't really able to indicate what could be happening other than maybe overwork or something. However, in her molecular U-test this is where we had the ability to look into it and we saw that there were quite a number of biomarkers that I know from my biochemistry background that these are indicators of cancer environment, like a tumor type of environment, and several of these biomarkers were orders of magnitude higher than what we would expect in the normal range for a healthy individual. And this is what really flagged for us that hey, something is really going wrong here. And taking a deeper dive into it, we noticed that these biomarkers are related to cancer. And taking a deeper dive into it, we noticed that these biomarkers are related to cancer and there were a number of. You know the thing with biomarkers as well is they follow a certain path of disease mechanisms that may overlap with different diseases. So we noticed that, you know, with pancreatic cancer being one of the risk factors, there was also ovarian and breast that may also be a risk factor as well.
Ash Anwar:
So we relayed this information to her doctor to say that, hey, we're seeing, you know, cancer biomarkers that are relevant in her profile and you know, kudos to her doctor. He was very receptive to this information. He spoke to her. There's a family history that that she had with a sibling who passed away from ovarian cancer, and so the doctor decided that, hey, we're gonna do a ct scan to see what's going on. And that's when they found the, the lesion, uh did a biopsy, and and that's when, after doing the biopsy, the lesion, they noticed that the biomarker for pancreatic cancer was a thousand times higher.
Ash Anwar:
And they were like, okay, this needs to be surgically removed. Luckily it was at a stage one where the cancer hadn't became aggressive yet, and so they were able to remove it just through surgery. No chemo was needed to be done and it was just after the surgery recovery. That's all that she needed to do. Now she's doing fine and we've done follow-up tests to also verify that all these cancer associated biomarkers are now back within normal ranges. And now it's just monitoring. Now it's making sure that once an individual has had cancer, there's an increased risk of cancer coming again. So we just want to make sure we monitor her and anytime we see another signal or something we know, right away we can put her on that clinical path to getting that analyzed.
Craig:
Yeah, build the future of multi-agent software with agency A-G-N-T-C-Y. The agency is an open source collective building the internet of agents. It's a collaboration layer where AI agents can discover, connect and work across frameworks. For developers, this means standardized agent discovery tools, seamless protocols for interagent communication, seamless protocols for interagent communication and modular components to compose and scale multi-agent workflows. Join Crew AI, langchain, lama, index, browserbase, cisco and dozens more. The agency is dropping code specs and services, no strings attached. Build with other engineers who care about high-quality multi-agent software. Visit agencyorg and add your support. That's A-G-N-T-C-Y. Dot O-R-G. Join other engineers who care about building the Internet of Agents. Who care about building the Internet of agents?
Craig:
You know there are a number of companies that are offering, you know, screening, biomarker screening now, and it's difficult to know which really have the models capable of doing the analysis. Which biomarkers are meaningful? Eric Topol was I mean, I didn't had not spoken to you yet when I spoke to him but he was cautious about a lot of these companies because he wasn't confident that they're following the correct biomarkers. How, frankly, how do I mean, have you published a lot of this? I mean, how do people, how do you engender confidence, particularly after you know confidence, particularly after you know blood biomarker scandals in the past, that this is real.
Ash Anwar:
Yeah, yeah, no, of course, I think that's a very, very valid question. I mean, even here, us at Molecular U, we're only scratching the surface when it comes to biomarker testing too. I mean, there's thousands of biomarkers, if not hundreds of thousands of biomarkers, in our body and they're relevant to a lot of different degrees, and it's really about picking the biomarkers that we believe have the highest value, and even then it's going to have value only in specific, perhaps niche, areas of interest as well. We have published on a number of our studies that are looking at these biomarkers and models and predictions of disease, and a lot of this is also classified into different I would say different omics.
Ash Anwar:
A lot of the early companies had focused on genetic testing because that was a really hot topic, you know. Could we look at someone's genetics and be able to predict diseases that they are predisposed to down the road? And so a lot of this personalized medicine and precision medicine really bore out of that genetic testing. Unfortunately, you know, one of the things that we've seen with genetic testing is that there's a lot of misinformation with the genomic information as well, and, secondly, the genes themselves don't exactly like. Being able to predict disease from genomics alone is not a very strong way of going about this process. Someone could have a genetic predisposition, but that could potentially not be expressed and the individual may not end up having that disease.
Ash Anwar:
And so where Molecular U is really uniquely positioned is we actually don't do genetic testing?
Ash Anwar:
Is we actually don't do genetic testing? We are looking at the dynamic biomarkers in the blood that change as things progress, just naturally through healthy aging, but also through any of the stressors or any life events that an individual is having as well, and these are the biomarkers that would directly affect the mechanisms that govern health and disease. The other aspect of molecular that is, I would say, uniquely positioned is that the biomarkers we're looking at first of all is a very I think we have the largest panel of biomarkers that we are looking at, but we also have an active scientific research team where we're constantly surveying the literature to identify any new biomarkers that are of significant interest and high value and then working towards experimentally bringing that into our panel to incorporate and boost up our ability to make predictions on a number of different chronic diseases as well. But again, I'm not going to say that we're catching everything, because there's a lot of areas that I'm sure we have gaps. We're working towards identifying and being able to fill those gaps as well.
Craig:
Yeah, when was Molecular U? I'm sorry if you've already said when was it founded.
Ash Anwar:
I believe it was founded in 2014, 2015.
Craig:
I believe it was founded in 2014, 2015. I see, and so you have an early cohort that you've been tracking since then. Yeah, right.
Ash Anwar:
Yes, yes, how they're tied to various diseases. Yes, yes, and when we've been working, we call them the pathfinders, because they were the initial cohort that we used to help us validate a lot of our models or systems, and being able to measure the accuracies of what we're predicting and absolutely being able to measure the accuracies of what we're predicting and absolutely like being able to track them over such a long time and to see kind of the endpoints that have occurred has enabled us to retroactively go back and look at the data and understand the trajectories of the different combinatorial biomarkers that have resulted in different health outcomes for these individuals. So, yeah, it's been really great to be able to have access to that information and being able to almost, I would say, simulate what another patient with a similar profile biomarkers could be potentially along that track of disease.
Craig:
Essentially, yeah, what is the model? What are the models that you're using?
Ash Anwar:
Yeah, that's a great question. We have a number of different models that we use depending on the disease and the type of biomarkers that are associated with it. Essentially, it is like supervised machine learning, right? So we supervise machine learning classification model. We have biomarkers that enable us, as a signature or a set of features, to be able to classify whether a person is going to be in the class of healthy class group or are they aligning with the important features that we've identified in the biomarkers that put them in the class of disease? Essentially, the specific type of model that we use. Again, it can change.
Ash Anwar:
Support vector machines is something we use quite often. We have a publication around that where we've used support vector machines to develop a model for cardiovascular disease, to identify patients at high risk of coronary artery disease. So I've actually published that with the team back in. I can't remember the year, but 2019, 2018, perhaps around there, and so a lot of those models, as I mentioned, could be support vector machines. We will use neural nets as well and as the machine learning models, or AI, has become more and more sophisticated, we've adopted more and more of the more cutting edge tools to see if we can enhance the prediction of the models.
Ash Anwar:
Can we make the model better? Can we incorporate other data inputs as well? Currently, the team is looking at incorporating not only so. At the current moment, we ingest a lot of information from the scientific research literature, which is constantly increasing every year. I think there's more than millions of papers that are published every year. So we have an AI engine that's able to scour all the scientific and clinical literature and pull out the key pieces of information for our scientists to evaluate and consider incorporating into the model. So that's one data input that we use.
Ash Anwar:
Second data input that we use currently as well is this real-time patient data. As we go through patients, they input their information as they go through their longitudinal health journeys. We're able to use that information as well, that data, to help make our models better as well. But especially this year, the third aspect of data that we're really looking forward to is how do we integrate other data inputs? Can we look into integrating electronic health records into our data, things that the clinics are routinely taking a note of every time they see a patient, whether that's doctor's notes, electronic health information, perhaps in the future, wearables as well? But this is where things become a little bit complicated because there is a lack of data standardization when it comes to information and wearable information. So that's that's a big challenge for for us right now to to incorporate the data. But it's kind of an active area of research and the more modalities we can put into the the models.
Ash Anwar:
Hopefully that will enhance our ability to to predict this even better.
Craig:
Yeah, and the. You know, supervised learning takes an enormous amount of labeled data to train an accurate model. What were the data sets that you started with?
Ash Anwar:
with. The data sets we started with are exactly what you said labeled data sets that usually accompany a lot of the published information too, so definitely the deep scientific literature, clinical literature. We're able to acquire the data sets of patients either from the authors or the publications that have used this, and a lot of biobanks will also have this information available as well. So being able to use that data as a basis to kind of develop the models and then also battle test this and see how accurate the models are at predicting disease as well with validating through real patients.
Craig:
Yeah, and where are you on the product journey? Are you has this? Is this fully productized? Is it still in the research stage?
Ash Anwar:
Yeah, we have. We have a product that is out in market right now and we believe has a good product market fit, especially with clinics that are and institutions that are looking at deploying this in a longevity and wellness kind of space. We also work with a lot of the product is also very useful for clinics that are in I would say call them specialty clinics as well. We work with. We've done work with neurology clinics, for example, where they have patients that have certain varying degrees of cognitive decline and they want to do an analysis to understand is it a cognitive, perhaps a cognitive impairment that is coming through, a nutritional issue or a metabolic issue, or is this something along the lines of a neurological component, like Alzheimer's disease? So our product is developed as well for these specialty clinics that want to look at specific niche disease areas as well.
Ash Anwar:
So we do have a good product side that is able to work B2B with the clinics. We do have a D2C angle as well so patients, if they're interested, are able to directly order the tests and get the results and have an actual platform that they're able to look at all these biomarkers, understand what these biomarkers mean for their health and trajectories for different diseases as well, and this is the very commercial side of molecular you. Where I'm also really actively involved is this very deep research side of molecular you as well and that really feeds into the product right. As we develop things on the research end and validate it, we can actually feed it into the product and into the commercial space. But the research side of it is really involved heavily with a lot of academics, for example, that are involved in research into different diseases, looking at novel biomarkers, and we provide our ability to our panels to look at these, but also our machine learning and AI capabilities to help them ramp up their modeling efforts on the research side as well.
Craig:
Yeah, as I said, I've seen a lot of companies doing similar things. Who do you regard as your biggest competitor?
Ash Anwar:
It's a good question, very good question. Competitor. It's a good question, very good question. Function Health, I would say, comes to mind. They've just closed a big round as well and, if I'm not mistaken, I think are valued in the billions of dollars. So I think Function is probably our biggest competitor. The unique differentiator, again with molecular U, is that function focuses a lot on a lot of standard clinical tests that are routinely available to doctors already. However, they're able to take those standard tests and combine them and come up with these health areas of interest that the clinicians find useful as well. Where molecular U is very uniquely positioned is we go much more beyond just the standard clinical tests and look at the plethora of these biomarkers that are more pre-diagnostic and really giving you that early indicator of disease before things are going awry. But yeah, function, I believe, is our, our. If I was to pin my hat on our competitor, it would be function, health yeah, and then uh, uh, where are you in in funding?
Craig:
I mean, you mentioned function, just had a big round. How much have you guys raised? It seems like there'd be a lot of money for this kind of activity.
Ash Anwar:
Yeah, no, absolutely. I think we're in the midst of our Series A right now. In terms of the actual number, I don't know the actual number that we've raised, but I can say that, comparatively to what Function has managed to close on, I would say that investors looking at our technologies are getting a significant discount in terms of the potential for where we're at a look, and they're free to contact our uh our, I guess, investor line, uh from molecular you to get more information on exactly how much we've raised and what our you know performance looking at right now yeah, um, so the research you're doing is to try and refine or curate the number of, uh, curate the number of biomarkers that you track.
Craig:
Is that right or is there?
Ash Anwar:
something else going on. No, the research is to yeah, it's to curate and identify novel biomarkers that we want to incorporate into our platform. We are also constantly evolving the different disease areas and biological mechanisms that we're including into our platform as well. Uh, the the information, the algorithms that make it into our commercial product need to be vetted and validated to a high standard before we put them in, and this is where the research aspect of it is is very key. Uh, a good example is that pancreatic cancer case study, which we did right, and it is solely in the space of of the research side of, because that's where we're able to really deep dive into that information and understand and actually being able to predict that pancreatic cancer stage one diagnosis.
Ash Anwar:
However, the cancer signature is not something we readily put into our commercial product for clinics and DTC.
Ash Anwar:
This is right now.
Ash Anwar:
Cancer is really a complex subject and it's something that we take with all seriousness, and we want to have an ability to really make sure that we are providing that best information we can on that cancers, but also, we don't want to scare patients as well.
Ash Anwar:
We want to make sure you know they're able to look at that information and understand it in the context with a clinician to to understand what the risks actually mean. So cancers is something that is not currently in the product right now, but it is something we're working on to actively put into the product within this year as well, and it's just something we really cognizant of. The patient journey, too right. We want to understand the work with our clinic partners and our patients as well, who trust in the science and the work that we're doing in a way to give them the transparency and the best information possible as well. So this is where the research side of things is really almost as a as a big sieve, in a way of doing a lot of innovative work but only giving information that is highly validated, highly transparent, accurate and evidence-based into towards our clinics and patients.
Craig:
Yeah, yeah. And on the case of the woman whose pancreatic cancer was caught at stage one, the things that were changing were not specific to pancreatic cancer. You could just see that there was something going on. And is that because the cancer was affecting other organs or other processes in the body?
Ash Anwar:
Correct, correct, yeah. So a lot of the biological processes that occur with cancer are conserved with other biological processes for other diseases as well. So, for example, with the pancreatic cancer, the biological processes or the disease mechanisms we're seeing were things like angiogenesis, which is the development of new blood vessels, for example. So in that case we were seeing a significant rise in the biomarkers associated with new blood vessel development. In the context of cancer that makes a lot of sense because the tumor wants to grow and is going to hijack the system to be able to get as much nutrients and resources to itself so that the tumor can grow. So angiogenic biomarkers are a big signal for that cancer disease mechanism. However, if you're somebody who is training for a marathon or working out really heavily, well guess what? Your angiogenic biomarkers are going to be up because you are in the active process of building muscle and so your normal biological system is going to increase those levels of angiogenic biomarkers to develop the new blood vessels to feed your increased muscle growth as well. So looking at just individual biomarkers or just looking at individual biological pathways is not going to be enough to give you that differentiation between what is actually happening or whether that's a disease process.
Ash Anwar:
In the case of the individual pancreatic cancer, that was one of the biological factors that we were seeing, one of the pathways that we were seeing, but along that we also saw metabolic dysfunction.
Ash Anwar:
We also saw changes in the extracellular matrix so that's where the cells lie on the surface and the changes in the extracellular matrix so that's where the cells lie on the surface and the changes in the matrix allow the cells to actually round up, and so this is just the stage of metastasis, where they're able to disconnect from the matrix and start being able to, you know, migrate or metastasize to another area and then start growing as well. So it's the multitude of signals that have allowed us to again not signal cancer directly, but almost to have the signals that say that in this patient, the environment is highly conducive to cancer. And this is where I think we really supplement the medical system, where the clinician was able to now be like okay, I'm going to investigate by using cancer diagnostic tools to understand what stage this is happening, and so that was a very good success story for us in that regard.
Craig:
Yeah, and the one test is not going to tell you that much, right? Just as you said, if you're training for a marathon, maybe some biomarkers will be out of normal range. It's really doing it over time. And how often do you guys recommend that patients who are tracking biomarkers have their blood sampled?
Ash Anwar:
Yeah, if they're actively changing habits and, you know, training for a marathon, for example, health optimization every six months is a really good turnaround to see how quickly the biomarkers are shifting and, as I mentioned, an annual checkup is probably something that most individuals would probably offer as well Individuals who like to essentially hack their biology right, Biohackers that are really interested in understanding and optimizing their health and saying that, okay, I'm going to make this change this month. I'm going to start working out. I want to know what effect is happening Anywhere between every three months to six months enables them to get much more precise windows into their changes that I'm making in terms of their health and being able to get that much more resolution into these biomarkers and trajectories as well, which would be my recommendation.
Craig:
Yeah, and so you guys are. The products are fully launched, but you're concentrating on B2B. I'm sorry that's what it sounded like, but consumers can what? Log on to molecular you and buy a test, or how does that work?
Ash Anwar:
Yeah, absolutely. So we are focusing on with clinics, because it is something that I think has been really beneficial to be able to work with health practitioners that are interpreting a lot of this information and they have the knowledge and understanding to be able to translate a lot of this information, and they have the knowledge and understanding to be able to translate a lot of this information to the patients as well. But, yes, patients, individuals who are interested in getting the test themselves, don't have to go through clinic. They're able to log into our website, go to our online store and purchase the test directly. Go to our online store and purchase the test directly. If they're in the United States, they will get a kit that will come right to their door. They can schedule a phlebotomy blood collection with our partner, where they will come in to the individual's home and take the blood sample and then we'll get it shipped into our our labs to be able to analyze and get a report back to that individual okay, um.
Craig:
Is there anything I didn't cover that you think listeners should know?
Ash Anwar:
uh, that's a good question. Uh, I think the one aspect I would say as well is that we talked a lot about biomarkers in the science, about the early detection and things like that. One of the things that we also do here at Molecular U is the information that we have is also translated into actionable insights that an individual can take.
Craig:
Oh, that's right.
Ash Anwar:
Yeah, yeah. So being able to look at these very same biomarkers. They're heavily influenced by how we live our lives, so we also provide in our commercial, in our product. We also provide an action plan that details specific dietary actions, exercise and supplements that are specifically targeted towards these individuals' biomarkers that might be out of range. We're working on enhancing that action plan with a lot more information as well, perhaps looking at things like sleep patterns, perhaps integrating some wearable information, as I mentioned as well. But right now, the Lifestyle Action Plan really is targeted around optimizing diet, exercise and supplements as well.
Ash Anwar:
And the other thing I will mention about this is, I think a key thing that I would want people to know about this type of testing, about molecular U, is the ability to differentiate away from genetic tests. Is really important to myself that I believe that this is really important. I feel like with genetic testing, there is almost like we're able to to offset the accountability. You know what I mean With like genetic testing. It's like, okay, I have a you know family history of, you know this disease, you know diabetes or something, and oh, I have some biomarkers for genetic biomarkers for diabetes. Unfortunately, there isn't anything I can do about it. So you know you kind of shift that accountability away.
Ash Anwar:
But the thing is we actually have a lot of control over our disease and longevity and healthy aging and that, and I think what with molecular, we're really able to bring that science and data and ability to to live your best life at the fingertips of individuals and being able to see information, track that information, make targeted decisions about what you could do with the biomarkers in your body and how they're shifting, to make active decisions on your health and really take control and be able to make the correct, the correct changes. Be able to see how those changes are reflecting in real time and not just like you know, oh, my weight didn't change, I'm exercising, but nothing's happening with biomarkers. You actually see that effect, that beneficial, positive effect that's occurring and then being able to take that and and eventually lead to a really amazing like healthy aging longevity aspect as well.
Craig:
Yeah, Well, that's fascinating Uh.
Sonix is the world’s most advanced automated transcription, translation, and subtitling platform. Fast, accurate, and affordable.
Automatically convert your mp3 files to text (txt file), Microsoft Word (docx file), and SubRip Subtitle (srt file) in minutes.
Sonix has many features that you'd love including transcribe multiple languages, world-class support, upload many different filetypes, share transcripts, and easily transcribe your Zoom meetings. Try Sonix for free today.